
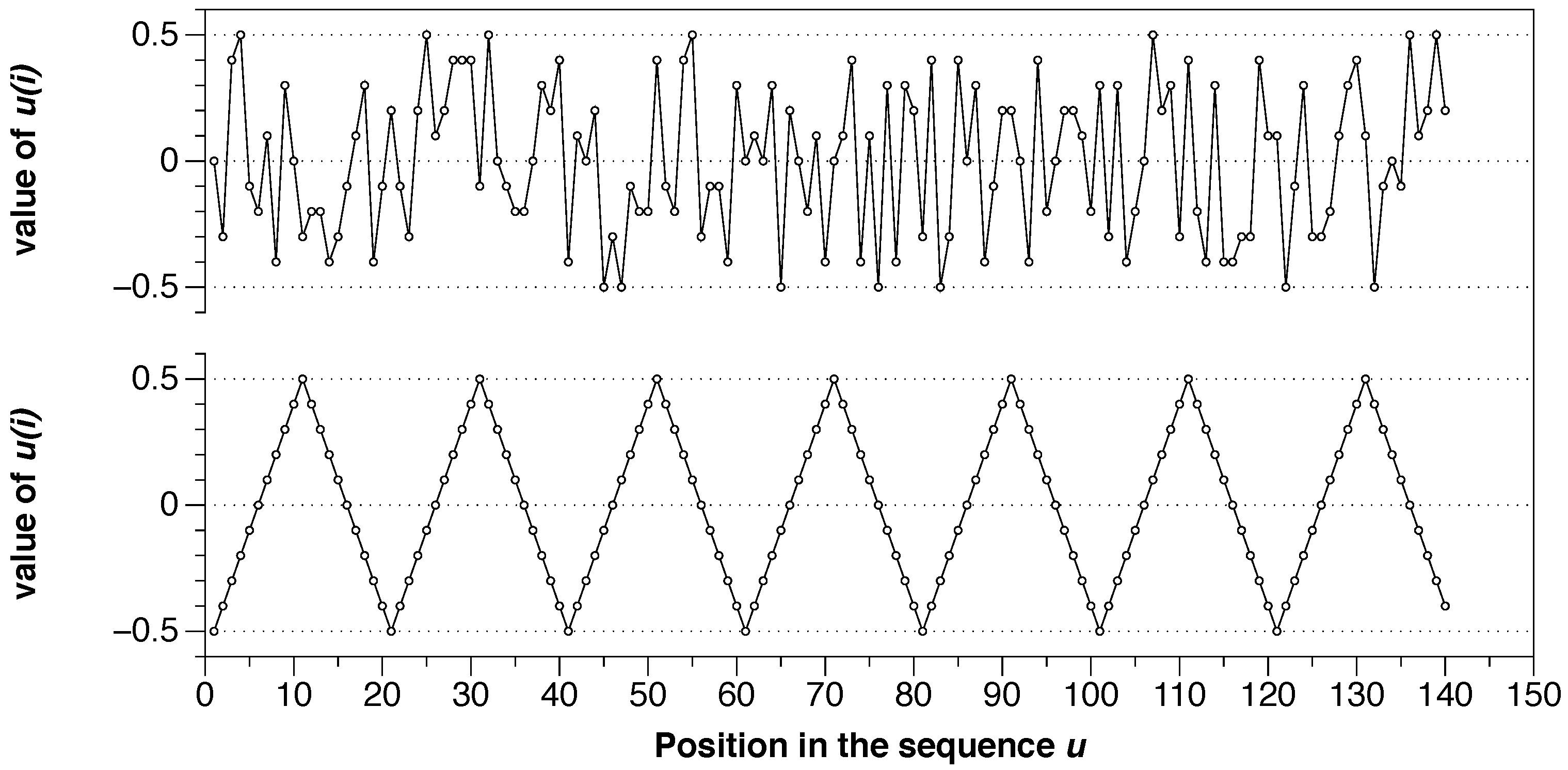
Software, TCS High Entropy Alloys Database v3 (2019).Ĭ. The correlations between the predicted composition, hardness, and microstructure are also discussed. This work successfully demonstrates that, by applying the machine learning method, new compositions of AlCoCrFeMnNi-based HEAs can be obtained, exhibiting hardness values higher than the best literature value for the same alloy system. Without any guidance, the design of new compositions of AlCoCrFeMnNi-based HEAs would be difficult by empirical methods. (b) Annual publications retrieved with the keywords machine learning material and machine learning high-entropy alloys (inset plot) from ScienceDirect (blue) within the subject area of materials science, Nature Portfolio journals (green), and Physical Review journals (red). Machine learning approaches require large annotated training data to estimate and smooth model parameters. Machine learning approaches to NER include HMMs, CRFs, support vector machines (SVMs), and maximum entropy models. A bootstrap approach is adopted to quantify the uncertainty of the prediction. Rule-based systems construct rules manually or automatically and use them for entity detection. A simulated annealing algorithm is integrated with the ANN to optimize the composition. The combination of multiple-principal element materials, known as high-entropy materials (HEMs), expands the multi-dimensional compositional space to. This work utilizes an ANN to predict the composition of high-entropy alloys (HEAs) based on non-equimolar AlCoCrFeMnNi in order to achieve the highest hardness in the system.
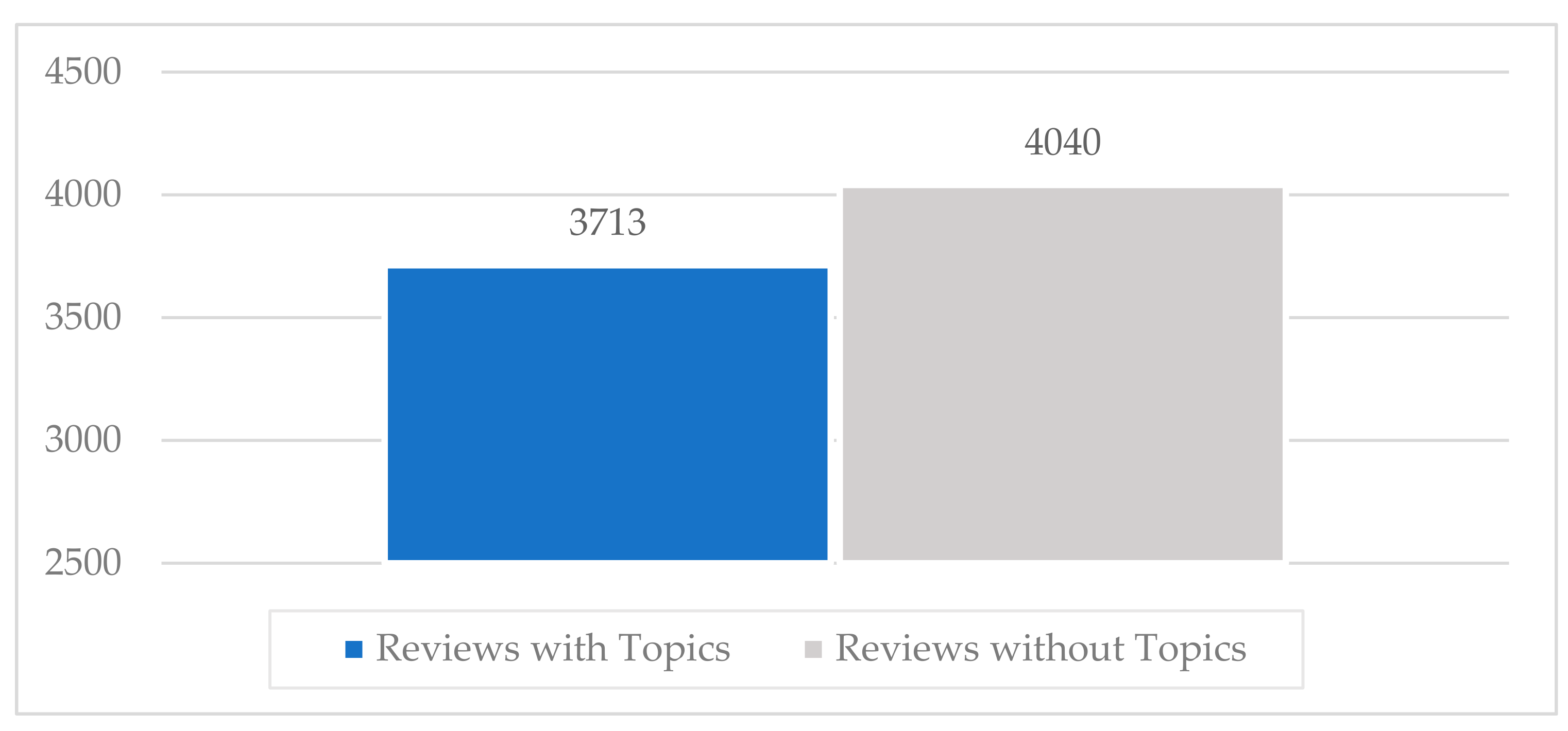
Machine learning with artificial neural network (ANN)-based methods is a powerful tool for the prediction and exploitation of the subtle relationships between the composition and properties of materials.
